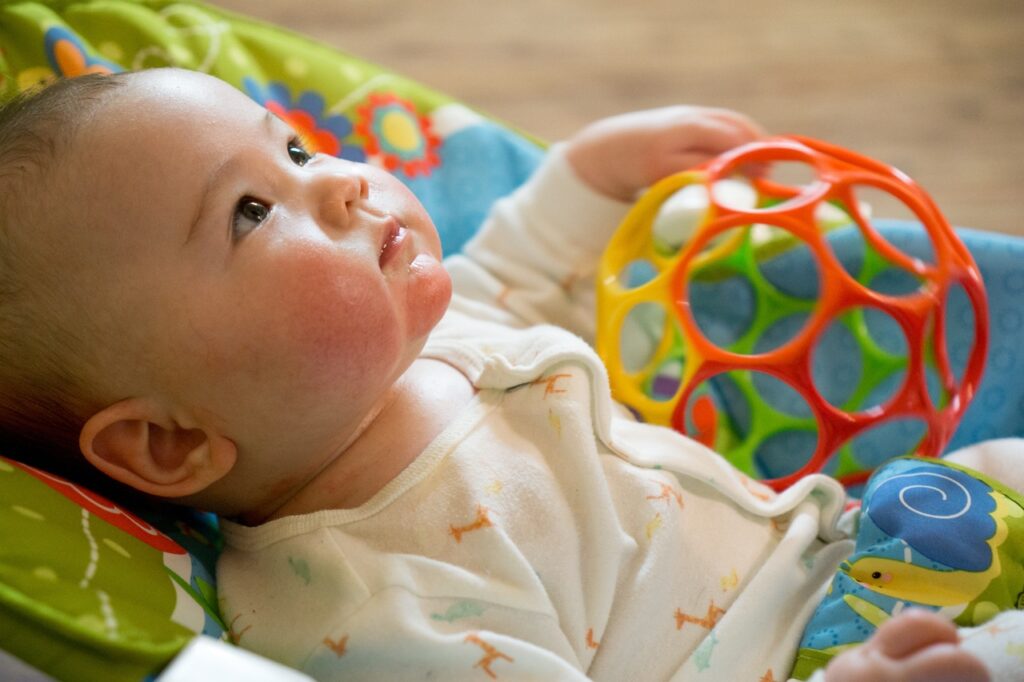
Baby focusing on visual stimulus. Photo: Pxhere
Babies are amazing.
Their brains, which begin life with around 100 billion neurons and only a fraction of the 100 trillion connections that will eventually form, develop with impressive speed. They start to learn how to hear and walk, to figure out feelings, and develop problem-solving skills with the help of adults to model behavior. With loving adult care, teaching, and stimulation, the infant’s brain wires up at an incredible rate of 700 new connections per second.
“For the last 15 years or so computer scientists and developmental cognitive scientists have been trying to figure out how children learn so much so quickly, and how to design a machine that could do the same,” says Dr. Alison Gopnik, Professor of Psychology and Affiliate Professor of Philosophy at the University of California at Berkeley. In 2019, she argued in The Wall Street Journal that “The future of artificial intelligence depends on designing computers that can think and explore as resourcefully as babies do.”
Engineers at DeepMind, which is owned by Google’s parent company Alphabet Inc., have followed this reasoning to build a machine-learning system based on what we know of how babies’ brains work. In a 2022 paper published in the journal Nature, Dr. Luis Piloto, a neuroscientist and cognitive scientist at DeepMind since 2016, co-authored a report on an experiment in which kernels of knowledge on physics that babies seem to know intuitively from birth were input to a machine learning system.
Normally, AI can easily beat humans in games such as chess and poker, and can quickly generate calculations for practical or theoretical use.
These are tasks that we, as adult humans, label as hard. However, for machines, the real difficulty lies in tasks researchers refer to as “intuitive physics.” “’Intuitive physics’ enables our pragmatic engagement with the physical world and forms a key component of ‘common sense’ aspects of thought. Current artificial intelligence systems pale in their understanding of intuitive physics, in comparison to even very young children,” the paper states.
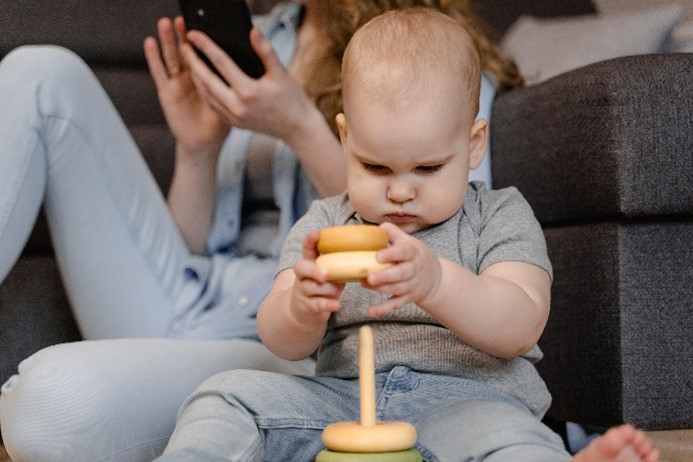
Block play promotes the development of spatial awareness and develops hand-eye coordination (Teach Early Years). Photo: Mart Production
Dr. Piloto and co-authors provide detailed examples of these intuitive physics concepts, and how they seem simple enough for babies. For instance, there’s the concept of solidity, and how babies “expect that objects will not interpenetrate one another.” Another is continuity, as babies already “expect that objects will not magically teleport from one place to another but instead trace continuous paths through time and space.”
Cognitive psychologist Dr. Susan Hespos, from the Infant Cognition Lab at Northwestern University, who holds a Ph.D. in developmental psychology, explains yet another example of intuitive physics noticed in babies: “boundedness.” An example of boundness, she explains, is “when you pick up your coffee cup, it sticks together. You don’t end up with just the handle.”
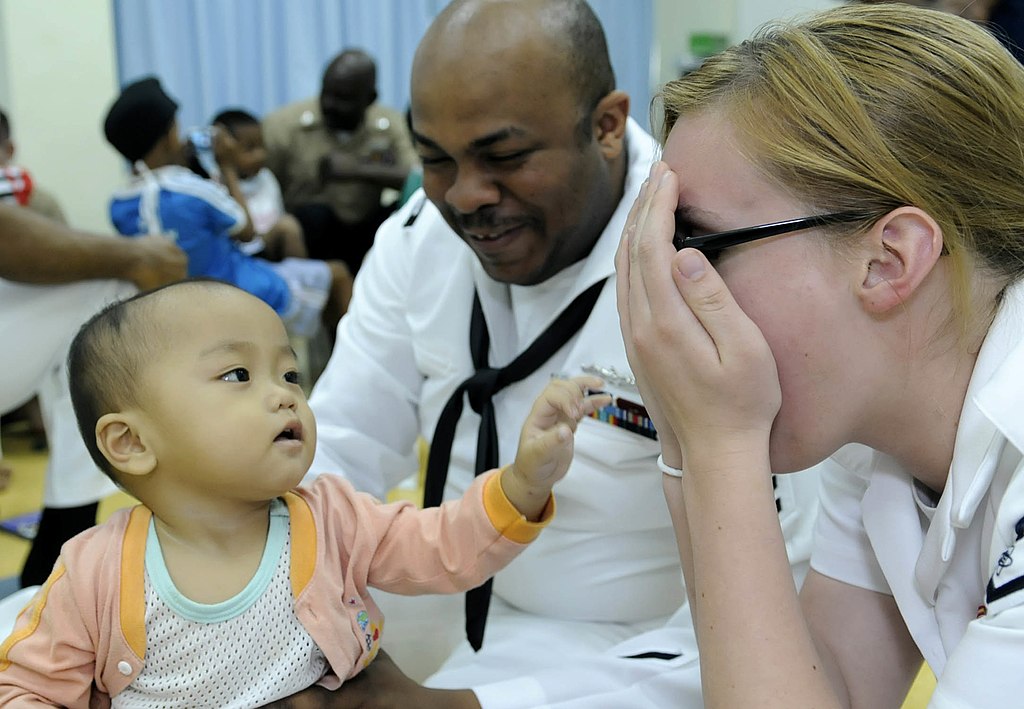
Adults playing peek-a-boo with a child, a game that helps develop object permanence, or continuity. U.S. Navy/Cynthia Griggs
Artificial intelligence models usually begin with a blank slate and are trained on huge data sets, learning from an enormous amount of cases.
Scientists have questioned whether this is the reason why babies outsmart machines in cognitive tasks that adults usually take for granted. Babies appear to have a set of expectations about objects that is built into their brains. The researchers showed that modelling a deep-learning AI system based on what babies know allows the machine outperform AI systems which begin with nothing and try to learn based on experience alone.
The software model, named Physics Learning through Auto-encoding and Tracking Objects (PLATO), is based on ‘object-centered coding’ inspired by infant cognition. The system was trained on hours of videos and learned patterns such as continuity, solidity, and persistence of the shape, or boundedness, of objects.

Although machines outperform adults in many games and tasks, babies are better at intuitive physics. Mike MacKenzie
“In an object-centered representation, the position of the subparts of an object are encoded with respect to a set of axes and an origin centered on the object. Several physiological and neuropsychological results support the existence of such representations in humans and monkeys,” explain Dr. Sophie Deneve and Dr. Alexandre Pouget.
As explained in the article in Nature, “Developmental psychologists test how babies understand the motion of objects by tracking their gaze. When shown a video of, for example, a ball that suddenly disappears, the children express surprise, which researchers quantify by measuring how long the infants stare in a particular direction.”
In their experiment, Dr. Piloto and co-authors found a fascinating, albeit predicted, result: PLATO outperformed usual AI systems in the selected tasks. When shown videos with ‘impossible’ events, the machine showed ‘surprise’ as a baby would when faced with an object suddenly disappearing. While surprise in infants is measured by duration of their visual gaze, the machine’s surprise was computed based on the model’s prediction error.
For Dr. Hespos, “the research is a step towards making machine learning systems more efficient thinkers — like humans. Even the tiny ones.”