This YouTube video by 3Blue1Brown illustrates the operation of machine neural networks in layers, with components activated by weightings of inputs.
By James Myers
Machine “learning” is powered by neural networks, so called because of the similarities in the interconnections of these information circuits to the way that over 86 billion neurons in the human brain connect with each other.
The operation of machine neural nets is similar to the way different cells in the human eye work together to create an entire scene. For example, some eye cells are responsible for identifying the edges of objects, while others are tasked with perceiving depth and colours in wavelengths of light. Neural nets employ huge numbers of similar connections to assemble a complete set of information and apply it in a given context, in a process that has been called “learning.”
Neural nets have rapidly advanced and have impressive capabilities. The public introduction of GPT-3 technology in November 2022 by OpenAI, and its upgrade to GPT-4 in March 2024, has sparked much discussion about the power and limitations of the technology. While the neural net underlying GPT-4 can produce impressive human-like outputs, it can also make significant errors which have been termed “hallucinations.”
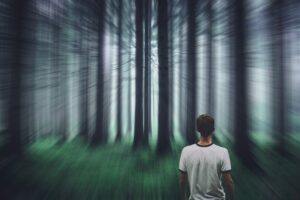
Imagine all of the data that comprise an entire scene, assembled in the human eye. Image by ELG21 from Pixabay
Recently, two lawyers in New York were fined for using GPT technology to produce a court filing for an aviation injury lawsuit. The filing contained six apparently realistic but fictitious case references that, for unknown reasons, the technology invented. Either not understanding the machine’s limitations, or not wanting to spend the time to verify its outputs, the lawyers failed to fulfill their professional responsibility to prevent such “hallucinations” from contaminating the legal record.
While OpenAI openly advises users of the imperfections in the company’s technology, we might consider reinforcing our understanding of the differences between the neurons in our brains and the connections in the machine.
To begin with, we could use different language to describe the machine’s functions. For example, the machine does not “learn” in the way that humans learn from each other, and the machine does not perceive meaning in the way that humans appreciate meaning in the context of lived experiences. Further, machines “malfunction” while a human might “hallucinate.”
The “neurons” in the machine are not comparable to the neurons in human brains, and so maybe we could find another word for the machine networks. For instance, as neuroscientist David Eagleman explains, each human neuron has the entire human genome in it and there are half a quadrillion connections among our 86 billion neurons. Human brains have neuroplasticity, which means that they can “re-wire” the connections of neurons and synapses under changing conditions, a reprogramming feature not found in the machine. In fact, a damaged human brain can sometimes recover its full operation by redistributing key functions to its healthy sectors, while a damaged machine requires a human to fix it.
Ultimately, a key difference between machine neural nets and the neurons in human brains is imagination.
Human neurons are capable of imagination, a facility we have used over thousands of years and which transfers from generation to generation. The human imagination is in evidence everywhere on this Earth in the cities we have built, waterways we have diverted, roads we have constructed, and technology we have invented. While machines can execute algorithmic instructions to create sophisticated images and outputs, these are not the products of any imagination on the part of the machine.
As many commentators have pointed out, the powerful neural net underlying GPT technology can only predict an output based on a human input. It does this by continuously applying and adjusting weightings to probabilities until an output “fits” the dataset on which it was “trained.”
There is, however, a time limitation for such machine training datasets, which skews the weighting of its probabilities. While OpenAI hasn’t disclosed the most recent date of information included in GPT-4 data, the data will certainly not be up to the minute. For instance, when GPT-3 was released, its data was over a year old.
For the machine, time has no meaning, but for the human it does. If a cure for cancer were discovered yesterday, machines trained on older data would not know it – although clearly news of such a momentous and meaningful event would quickly distribute between humans.