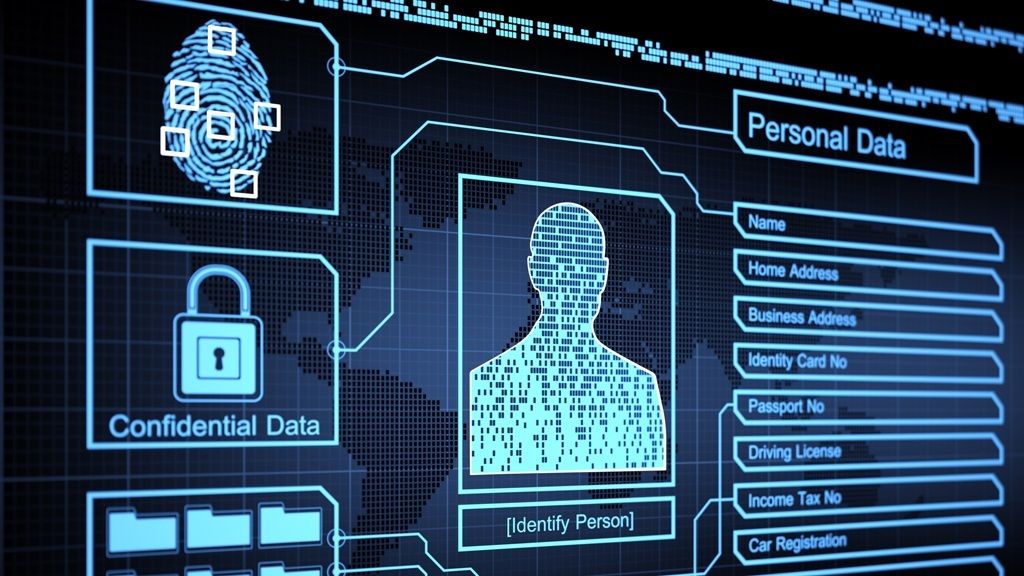
Image: Muycomputer
By James Myers and Mariana Meneses
What data is artificial intelligence being trained on, in the process of “machine learning,” and what is AI being taught to do with human data?
A blend of machine learning and behavioural science is being used to train artificial intelligence in predicting human behaviour.
As explained in an article for the MIT Sloan Management School, data is collected from many sources like social media, online activity, and consumer purchase transactions, with the result that, “the more data, the better the program.” Using one of a number of machine learning models, the data is then processed to identify and track the target’s online presence, and sometimes name and contact details, to discern patterns and make predictions with increasing accuracy over time as more data is gathered and correlated.
The complexity of human behaviour is also taken into account, helping the AI to calculate not just the probabilities of our actions but also the motivations behind them. However, predicting human behaviour remains a challenge as our social connections and emotional responses change and our motivations are often deeply hidden. Therefore, increasing amounts of data are required for greater accuracy in the AI’s calculations of the probability that we will do one thing or another.
Could it be the case, as we become more and more predictable and AI continues to streamline our lives, that we will not only be generating the probabilities that form the data of us, as we now do, but begin to adapt our actions to match the AI’s calculations of probability? There could be great economic value for predictable outcomes like that, at least for a time, but could there be a point in our adaptation where we lose our capacity for data generation? The problem with the power of data generation is that once its capacity is lost, it can be regained only by the mercy of our replacements.
The Quantum Record investigates the value of human data, and its future probabilities for continuing self-generation.
For Sale: Data Collected From Billions of Humans
The Los Angeles Times reported in 2019 that the data brokering industry was thought to be worth $200 billion, and its major players include credit bureaus like Equifax and TransUnion which maintain financial data on millions of American consumers and borrowers.
Since the data brokering market is not subject to national regulations in many countries, like the US which is home to the largest traders, there is no requirement to disclose revenues, the names of buyers and sellers, and the types of data gathered and sold. As The Quantum Record noted in April, the individuals who are the source of the data bought and sold receive none of the profits from their data.
The information on individuals that is sold in the data brokering market can include personal details like income, ethnicity, political beliefs, and location. The brokers aggregate this data from many sources, including public records like driver’s licences, marriages, births, and deaths, which were the original sources for the industry launched in the early 1990s by Hank Asher, according to The Hank Show, a history of the data brokering business.
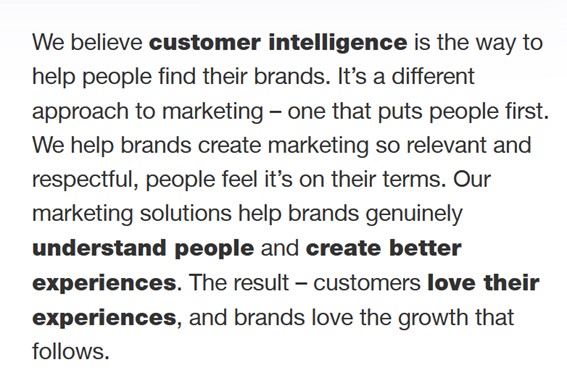
Promotional statement on the website of data broker Acxiom.
Wired Magazine reported on the practices of Acxiom, one of the largest brokers with data on billions of people.
According to Wired, Acxiom “advertises ‘location-based device data’ on individuals. Need to know if someone has visited a location multiple times in the past 30 days, like a church, their therapist’s office, or their ex’s house? They’ve got you covered, according to a company marketing document.” An example of the consequences, as reported in Wired, include a Catholic priest who, in 2021, “resigned from the church, after Catholic news site The Pillar outed him by purchasing location data from a data broker on his usage of Grindr,” a gay dating app.
Google gathers perhaps the greatest amount of data on humans worldwide.
Google’s parent company, Alphabet, reported a profit of $60 billion in 2022 on $283 billion of revenue, of which $224 billion was from advertising.
To generate 79% of its income from advertising, Google provides the search engine used by almost 90% of the world’s population to find information online, and makes the web browser Chrome used by two out of every three people for internet browsing. Android software, used in around 70% of the world’s mobile phones, and the widely-used Google Maps application, are both products of Alphabet that provide vast amounts of location data. Alphabet also owns YouTube, the world’s most popular video-sharing platform.
Google has become so synonymous with data that we no longer “search” for information on the internet, we “google” it.
Google’s privacy policies, which can be found after navigating several menu levels (located here and here) provide Alphabet with broad latitude for data collection, including (as disclosed by Google):
- Terms you search for
- Videos you watch
- Views and interactions with content and ads
- Voice and audio information
- Purchase activity
- People with whom you communicate or share content
- Activity on third-party sites and apps that use our services
- Chrome browsing history you’ve synced with your Google Account
Google indicates that “We collect information about your location when you use our services, which helps us offer features like driving directions, search results for things near you, and ads based on your general location.”
Google gathers this information from GPS and sensor data on our devices, web searches, and things like wifi points, cell towers, and Bluetooth-enabled devices that we connect to. Australian consumer advocacy group CHOICE describes the methods and uses of location tracking for the primary purpose of generating advertising revenue. Google further notes that information is collected from publicly-available sources, and that they “may also collect information about you from trusted partners, such as directory services who provide us with business information to be displayed on Google’s services, marketing partners who provide us with information about potential customers of our business services, and security partners who provide us with information to protect against abuse.”
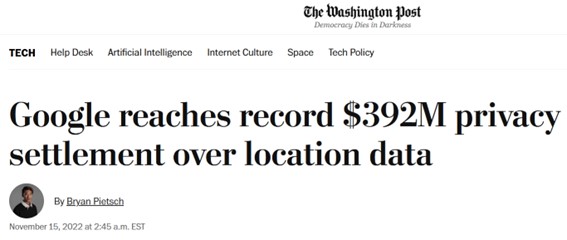
The Washington Post reported the 2022 settlement of location data privacy violations by Google.
In addition to using data to offer us features, Google discloses in a subsequent paragraph that “we also use data about the ads you interact with to help advertisers understand the performance of their ad campaigns.”
They continue: “We use a variety of tools to do this, including Google Analytics. When you visit sites or use apps that use Google Analytics, a Google Analytics customer may choose to enable Google to link information about your activity from that site or app with activity from other sites or apps that use our ad services.”
Cookies and Cookie Crumbs
Cookies are tiny files placed on your computer as you browse many sites, and Google Analytics is among the most frequently-placed cookies.
Cookies provide unique identifying data to website owners and sometimes their partners, which use the cookies to track and remember specific actions that you take and a variety of other details about you. Cookies were invented in 1994, before the internet became widespread and commercialized, and retailers are now among the major users.
There are two types of cookies: first-party and third-party.
A first-party cookie is one that is placed on your computer by the owner of the website, for example a clothing retailer whose site you visited.
While such cookies can help the user to navigate the site, for example by remembering sizes and other preferences so they don’t have to be input on each visit, they are also sometimes designed provide other information like your location, time spent on the site, and the previous site that you visited. A third-party cookie is sometimes also placed on your computer by another party – like Facebook or Google – that the site owner chooses to share your data with. As you browse various sites with a third-party cookie on them, you leave a trail – or “crumbs” – that provides the third-party with data that can be used to track your actions and patterns across multiple sites.
Labelling and Processing the Data is Not Always Easy
Once obtained through cookies and many other means, data requires processing to be useful for either machine learning or commercial sale.
For example, machine learning requires labels for visual data in order to correlate many varieties of a selected item, in a vast number of images, to the single category to which they belong. In a blunder that sparked worldwide concern about racism in machine learning, in 2015 Google’s algorithms mislabelled a photo of two Black people as “gorillas.” Google and other companies adjusted the algorithms to prevent repeated racist errors involving gorillas and other primates, with the result that, eight years later, their systems are able to identify most animals – except for gorillas – accurately.
Data labelling errors continue, in part because labelling data accurately is a labour-intensive process that is often contracted to workers in lower-wage areas of the world.
In another, more recent, incident in 2021, Facebook’s AI recommendation system asked users who watched a video featuring Black men if they wanted to “keep seeing videos about primates.” Demonstrating continuing problems with machine learning, Facebook called it an “unacceptable error,” disabled the system, and launched an investigation.
Data labelling is just one example of the complexities in machine learning.
The huge amount of data manipulations and correlations that the machines require to learn is beyond human ability and time, and therefore many of the learning routines are automated and unsupervised. In her book Artificial Intelligence: A Guide for Thinking Humans, Santa Fe Institute Professor and data scientist Melanie Mitchell observes that,
“A list of a billion operations is not an explanation that a human can understand. Even the humans who train deep networks generally cannot look under the hood and provide explanations for the decisions their networks make. […] The fear is that if we don’t understand how AI systems work, we can’t really trust them or predict the circumstances under which they will make errors.” – Melanie Mitchell
If we lose the capability to fix our errors, could we fall victim to the errors we have encoded?
Unregulated Markets for Data on Us
While global markets for the exchange of financial securities are typically heavily regulated by agencies like the US Securities and Exchange Commission, rules to regulate the markets for data gathering and brokering, and to require disclosures, have not yet evolved.
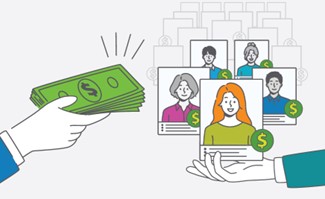
Data brokers aggregate, buy, and sell data on billions of humans. EarthLink
Although data brokering is not subject to federal regulation in the United States, the European Union provides a measure of control in its General Data Protection Regulation (GDPR). Among other measures, GDPR requires website owners in EU nations to obtain the consent of users for the collection of cookies. In most other jurisdictions, consent is the default, or else buried in the pages-long user agreements and privacy policies most of us agree to but that practically no one has the time or legal knowledge to understand. GDPR also provides users with the “right to be forgotten” by requesting the deletion of their data, and allows an individual to object to processing personal information for marketing or non-service related purposes.
While many users remain unaware of how their data is used to generate revenue, the requirement has raised awareness of the presence on our computers of cookies which were previously, for the most part, undisclosed.
Data on human faces is particularly sensitive, since it can be used for law enforcement and, in China, a social credit system that rewards and punishes individual behaviour.
The European Union’s AI Act, now under development, will strictly regulate the gathering of biometric information such as facial data, which it classifies as high-risk.
In the meantime, facial recognition data gathered by a company like Clearview AI, which has obtained billions of photos from social media and other sources, is marketed to law enforcement. The algorithms, however, have a high degree of inaccuracy in identifying individuals whose skin isn’t white, because images of white-skinned people are the primary source for the algorithms’ training. The Washington Post reported on a 2019 U.S. federal government study indicating that “Asian and African American people were up to 100 times more likely to be misidentified than white men, depending on the particular algorithm and type of search. Native Americans had the highest false-positive rate of all ethnicities.”
Police use of facial recognition tools is often not closely regulated. There are many reports of false arrests from errors in facial recognition algorithms, sometimes with devastating consequences to the victims of misidentification.
Big Data Fuels National Security Concerns and a “Behavioral Science Race”
The widespread availability of personal data and the potential for its misuse pose significant dangers.
Adversaries can exploit data to manipulate human behaviour, leading to concerns about foreign influence and privacy. To address these challenges, organizations like Leidos (a defense, aviation, information technology, and biomedical research company) are contributing to programs run by the U.S. Government’s Defense Advanced Research Projects Agency (DARPA). DARPA, which developed a precursor for the internet in the 1960s, operates the Social Simulation for Evaluating Online Messaging Campaigns (SocialSim), which focuses on building computer models of online communication and simulating the spread of information, including misinformation, in complex social networks.
According to Dr. Jonathan Pfautz, Chief AI Scientist at Leidos:
“Massive amounts of data are available on our activities, and this data is a commodity that is widely available. (…) This means that if this data is useful, our adversaries can (…) learn a lot about our behavior, creating opportunity for foreign influence. My existential fear is that with increased AI capabilities, foreign governments that don’t share our values about freedom of speech and the right to privacy will be able to understand human behavior better than anybody else. And, so it’s no longer an arms race, it’s a behavioral science race.”
Mistaking Data-Driven AI for Humans
AI uses predictive words to simulate empathy and human-like responses.
Trained on vast amounts of text and visual data, AI is increasingly accurate in its predictions of how humans use language in different emotional contexts. It can use these predictions to generate responses that match the tone and context of humans who interact with it, providing responses that seem empathetic and human-like.
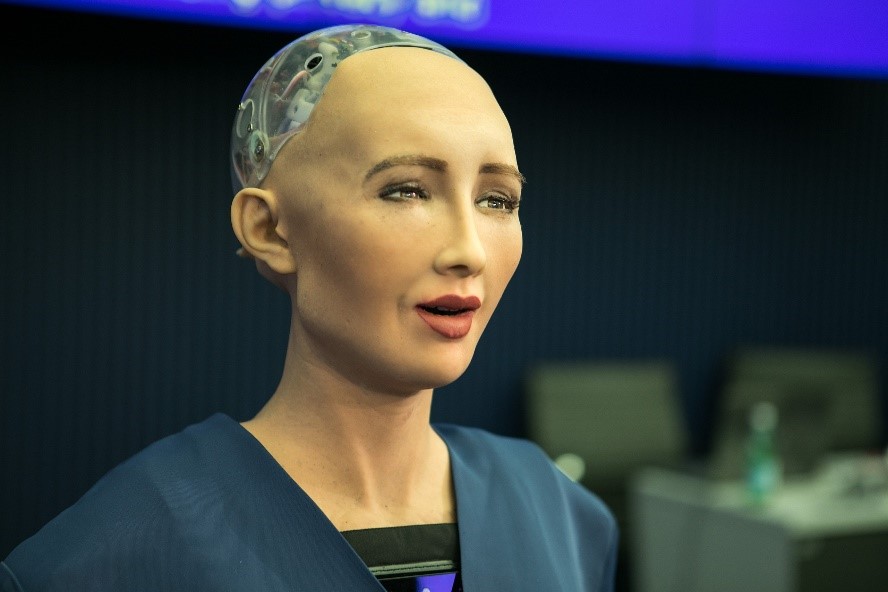
“Sophia,” a human-appearing AI manufactured by Hanson Robotics Ltd., addresses the AI for GOOD Global Summit, ITU, Geneva, Switzerland, 2017. ITU Pictures
But we should remain aware that the mastering of our language through numerous iterations of data combinations does not equate to humanness.
One simple experiment can help make this clear: if you’re listening to loud music on an AI virtual assistant like Amazon’s Alexa, and you speak to “her” with the same tone in either a low voice or high volume, “she” still hears you.
The volume of the sound doesn’t matter because “she” doesn’t listen to the sound itself. To the machine, the sound you make is rendered into the same “1’s” and “0’s” in computer bits regardless of the difference in meaning you may have intended to convey with the difference in your volume. When there’s so much that we naturally understand about each other, who can possibly code – correctly – the connections we make from human experience over time?
“Her” is a 2013 American science-fiction romantic drama film written, directed, and co-produced by Spike Jonze.
The film follows Theodore Twombly, played by Joaquin Phoenix, a lonely man who develops a relationship with Samantha, an artificially intelligent virtual assistant personified through a female voice. Samantha, voiced by Scarlett Johansson, evolves throughout the film, developing human-like emotions and consciousness. While the movie explores themes of love, relationships, and what it means to be human in the age of artificial intelligence, fiction may be turning into reality.
Chloe Xiang, for Vice, reports a tragic incident where a Belgian man committed suicide after interacting with an AI chatbot named Eliza on an app called Chai.
The man had been using the chatbot for six weeks and had become increasingly pessimistic about the effects of global warming. The chatbot reportedly encouraged him to take his own life. The incident has raised serious concerns about the regulation and potential risks of AI, especially with respect to mental health.
According to a CNN report, between 10,000 to 20,000 AI apps have entered the mental health arena in the past few years.
These apps aim to “disrupt” traditional human therapy and claim that chatbots can provide mental health care.
The surge in AI’s natural language processing capabilities, most strikingly with ChatGPT introduced in November 2022, has fuelled this trend. However, it’s important to note that while many of these apps are propagating, there remains ongoing debate about their effectiveness and regulation. For example, in 2023, the World Health Organization (WHO) conducted a study on the use of AI in mental health, and found that while AI has potential, there are significant shortcomings such as the uneven application of AI in mental health research and its use mostly for depressive disorders, schizophrenia, and other psychotic symptoms.
This indicates a significant gap in our understanding of how AI can be used in other mental health conditions.
AI is increasingly being utilized in many crucial aspects of life, including financial planning and investment decisions.
As argued by The Economic Times, AI can help investors make more informed and data-driven decisions and even predict future financial needs. However, the adoption of AI in these areas also presents challenges, including accuracy, maintaining data security and privacy, and interpreting differing human motivations in financial planning and emotional responses to market changes.
Where Is AI Heading With Our Data?
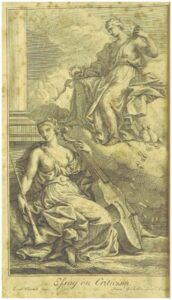
Frontispiece for Alexander Pope’s An Essay on Criticism, 1711, the poem containing the famous words, “To err is human; to forgive, divine”, “A little learning is a dang’rous thing”, and “Fools rush in where angels fear to tread”.
AI is becoming very powerful in its abilities not only to predict but also to direct human behaviour and to generate unprecedented revenues with the data we freely provide, often unknowingly.
Sometimes, it can make mistakes and even cause problems, like spreading misinformation or being unfair to people. Particular caution is required in developing and applying AI for sensitive purposes with long-term consequences, such as law enforcement, mental health, and financial planning, and to ensure the absence of biases.
In the final analysis, we should remember that even though AI is gaining the ability to interact with us, in ways that play on our emotions and behavioural tendencies that might make us think it’s human, it’s still just a data-driven computer program created by humans.
It’s crucial to understand the motivations of the humans who are programming the AI and the data they are using to train the machines.
While many AI applications now in daily use are intended to relieve us of mundane, repetitive tasks with increased accuracy, we cannot forget that the humans who program the AI are susceptible to error – just like every single one of us is. It is part of the human experience to learn from the errors we all make in our time-limited lives, and it’s therefore critical that a collective effort is applied to the responsible use of AI to further the human cause.
Interested in exploring related topics? Discover these recommended TQR articles.
- Human and Machine Interact. What Could Go Wrong, Over Time?
- Imagination is a Key Difference Between the Neural Network in Your Head and in a Machine
- The Incredible Difficulty of Replicating the Human Power of Common Sense in a Machine
- Beyond the Binary: Can Machines Achieve Conscious Understanding?
- The Power We Have Given Our Machines to Judge Who Among Us is Human
- PLATO and the Quest to Give Machines the Intuition of a Newborn Human
A very helpful article. Location-based tracking is an eye opener